-
Notifications
You must be signed in to change notification settings - Fork 57
Commit
This commit does not belong to any branch on this repository, and may belong to a fork outside of the repository.
Merge pull request #51 from Immortalise/main
add "Improving Generalization of Adversarial Training via Robust Critical Fine-Tuning" ICCV 2023
- Loading branch information
Showing
32 changed files
with
4,365 additions
and
1 deletion.
There are no files selected for viewing
This file contains bidirectional Unicode text that may be interpreted or compiled differently than what appears below. To review, open the file in an editor that reveals hidden Unicode characters.
Learn more about bidirectional Unicode characters
This file contains bidirectional Unicode text that may be interpreted or compiled differently than what appears below. To review, open the file in an editor that reveals hidden Unicode characters.
Learn more about bidirectional Unicode characters
Original file line number | Diff line number | Diff line change |
---|---|---|
@@ -0,0 +1,166 @@ | ||
# Copyright (c) Microsoft Corporation. | ||
# Licensed under the MIT License. | ||
|
||
data/ | ||
results*/ | ||
|
||
# Byte-compiled / optimized / DLL files | ||
__pycache__/ | ||
*.py[cod] | ||
*$py.class | ||
|
||
# C extensions | ||
*.so | ||
|
||
# Distribution / packaging | ||
.Python | ||
build/ | ||
develop-eggs/ | ||
dist/ | ||
downloads/ | ||
eggs/ | ||
.eggs/ | ||
lib/ | ||
lib64/ | ||
parts/ | ||
sdist/ | ||
var/ | ||
wheels/ | ||
share/python-wheels/ | ||
*.egg-info/ | ||
.installed.cfg | ||
*.egg | ||
MANIFEST | ||
|
||
# PyInstaller | ||
# Usually these files are written by a python script from a template | ||
# before PyInstaller builds the exe, so as to inject date/other infos into it. | ||
*.manifest | ||
*.spec | ||
|
||
# Installer logs | ||
pip-log.txt | ||
pip-delete-this-directory.txt | ||
|
||
# Unit test / coverage reports | ||
htmlcov/ | ||
.tox/ | ||
.nox/ | ||
.coverage | ||
.coverage.* | ||
.cache | ||
nosetests.xml | ||
coverage.xml | ||
*.cover | ||
*.py,cover | ||
.hypothesis/ | ||
.pytest_cache/ | ||
cover/ | ||
|
||
# Translations | ||
*.mo | ||
*.pot | ||
|
||
# Django stuff: | ||
*.log | ||
local_settings.py | ||
db.sqlite3 | ||
db.sqlite3-journal | ||
|
||
# Flask stuff: | ||
instance/ | ||
.webassets-cache | ||
|
||
# Scrapy stuff: | ||
.scrapy | ||
|
||
# Sphinx documentation | ||
docs/_build/ | ||
|
||
# PyBuilder | ||
.pybuilder/ | ||
target/ | ||
|
||
# Jupyter Notebook | ||
.ipynb_checkpoints | ||
|
||
# IPython | ||
profile_default/ | ||
ipython_config.py | ||
|
||
# pyenv | ||
# For a library or package, you might want to ignore these files since the code is | ||
# intended to run in multiple environments; otherwise, check them in: | ||
# .python-version | ||
|
||
# pipenv | ||
# According to pypa/pipenv#598, it is recommended to include Pipfile.lock in version control. | ||
# However, in case of collaboration, if having platform-specific dependencies or dependencies | ||
# having no cross-platform support, pipenv may install dependencies that don't work, or not | ||
# install all needed dependencies. | ||
#Pipfile.lock | ||
|
||
# poetry | ||
# Similar to Pipfile.lock, it is generally recommended to include poetry.lock in version control. | ||
# This is especially recommended for binary packages to ensure reproducibility, and is more | ||
# commonly ignored for libraries. | ||
# https://python-poetry.org/docs/basic-usage/#commit-your-poetrylock-file-to-version-control | ||
#poetry.lock | ||
|
||
# pdm | ||
# Similar to Pipfile.lock, it is generally recommended to include pdm.lock in version control. | ||
#pdm.lock | ||
# pdm stores project-wide configurations in .pdm.toml, but it is recommended to not include it | ||
# in version control. | ||
# https://pdm.fming.dev/#use-with-ide | ||
.pdm.toml | ||
|
||
# PEP 582; used by e.g. github.com/David-OConnor/pyflow and github.com/pdm-project/pdm | ||
__pypackages__/ | ||
|
||
# Celery stuff | ||
celerybeat-schedule | ||
celerybeat.pid | ||
|
||
# SageMath parsed files | ||
*.sage.py | ||
|
||
# Environments | ||
.env | ||
.venv | ||
env/ | ||
venv/ | ||
ENV/ | ||
env.bak/ | ||
venv.bak/ | ||
|
||
# Spyder project settings | ||
.spyderproject | ||
.spyproject | ||
|
||
# Rope project settings | ||
.ropeproject | ||
|
||
# mkdocs documentation | ||
/site | ||
|
||
# mypy | ||
.mypy_cache/ | ||
.dmypy.json | ||
dmypy.json | ||
|
||
# Pyre type checker | ||
.pyre/ | ||
|
||
# pytype static type analyzer | ||
.pytype/ | ||
|
||
# Cython debug symbols | ||
cython_debug/ | ||
|
||
# PyCharm | ||
# JetBrains specific template is maintained in a separate JetBrains.gitignore that can | ||
# be found at https://github.com/github/gitignore/blob/main/Global/JetBrains.gitignore | ||
# and can be added to the global gitignore or merged into this file. For a more nuclear | ||
# option (not recommended) you can uncomment the following to ignore the entire idea folder. | ||
#.idea/ |
This file contains bidirectional Unicode text that may be interpreted or compiled differently than what appears below. To review, open the file in an editor that reveals hidden Unicode characters.
Learn more about bidirectional Unicode characters
Original file line number | Diff line number | Diff line change |
---|---|---|
@@ -0,0 +1,105 @@ | ||
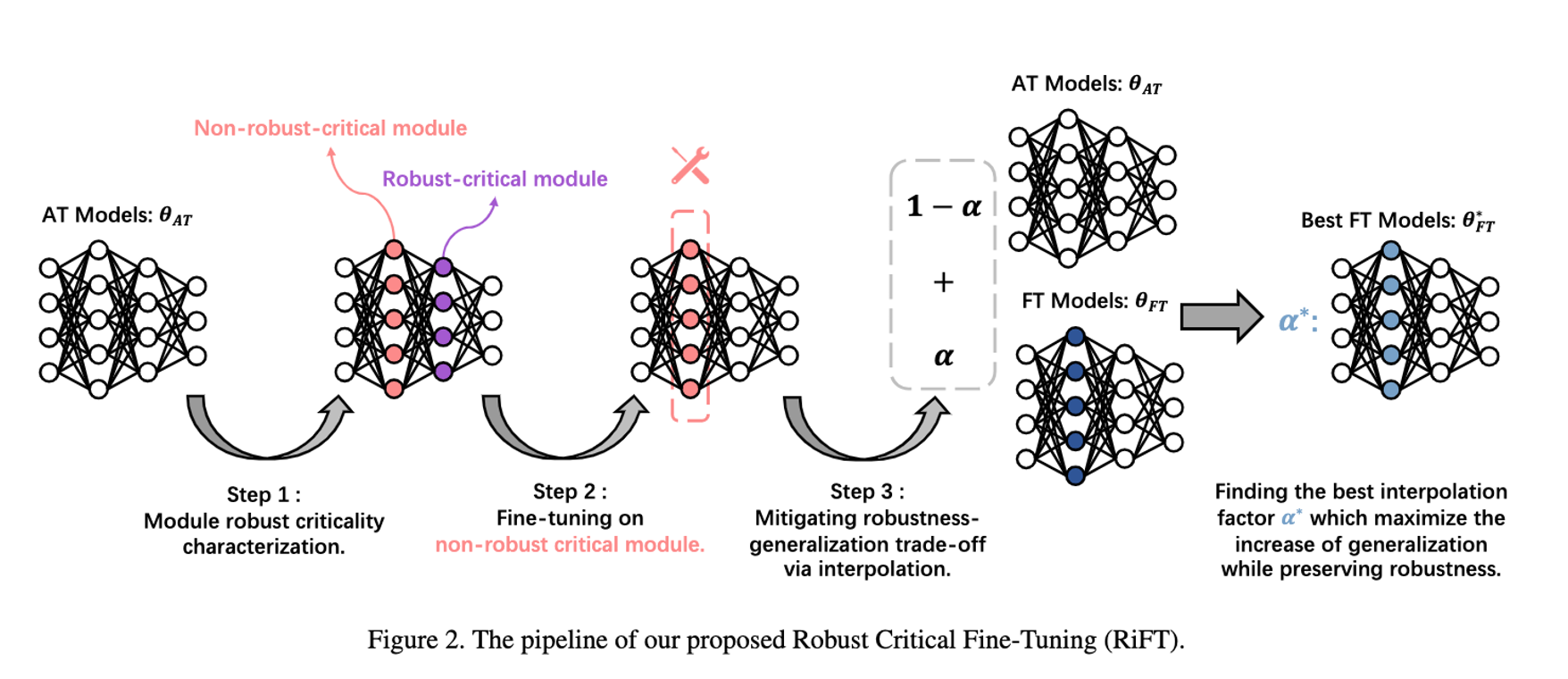 | ||
|
||
# Improving Generalization of Adversarial Training via Robust Critical Fine-Tuning | ||
|
||
This is the official implementation of ICCV2023 [Improving Generalization of Adversarial Training via Robust Critical Fine-Tuning](https://arxiv.org/abs/2308.02533). | ||
|
||
**Abstract**: Deep neural networks are susceptible to adversarial examples, posing a significant security risk in critical applications. Adversarial Training (AT) is a well-established technique to enhance adversarial robustness, but it often comes at the cost of decreased generalization ability. This paper proposes Robustness Critical Fine-Tuning (RiFT), a novel approach to enhance generalization without compromising adversarial robustness. The core idea of RiFT is to exploit the redundant capacity for robustness by fine-tuning the adversarially trained model on its non-robust-critical module. To do so, we introduce module robust criticality (MRC), a measure that evaluates the significance of a given module to model robustness under worst-case weight perturbations. Using this measure, we identify the module with the lowest MRC value as the non-robust-critical module and fine-tune its weights to obtain fine-tuned weights. Subsequently, we linearly interpolate between the adversarially trained weights and fine-tuned weights to derive the optimal fine-tuned model weights. We demonstrate the efficacy of RiFT on ResNet18, ResNet34, and WideResNet34-10 models trained on CIFAR10, CIFAR100, and Tiny-ImageNet datasets. Our experiments show that RiFT can significantly improve both generalization and out-of-distribution robust- ness by around 1.5% while maintaining or even slightly enhancing adversarial robustness. Code is available at https://github.com/microsoft/robustlearn. | ||
|
||
## Requirements | ||
|
||
### Running Enviroments | ||
|
||
To install requirements: | ||
|
||
``` | ||
conda env create -f env.yaml | ||
conda activate rift | ||
``` | ||
|
||
### Datasets | ||
|
||
CIFAR10 and CIFAR100 can be downloaded via PyTorch. | ||
|
||
For other datasets: | ||
|
||
1. [Tiny-ImageNet](http://cs231n.stanford.edu/tiny-imagenet-200.zip) | ||
2. [CIFAR10-C](https://drive.google.com/drive/folders/1HDVw6CmX3HiG0ODFtI75iIfBDxSiSz2K) | ||
3. [CIFAR100-C](https://drive.google.com/drive/folders/1HDVw6CmX3HiG0ODFtI75iIfBDxSiSz2K) | ||
4. [Tiny-ImageNet-C](https://berkeley.app.box.com/s/6zt1qzwm34hgdzcvi45svsb10zspop8a) | ||
|
||
After downloading these datasets, move them to ./data. | ||
|
||
The images in Tiny-ImageNet datasets are 64x64 with 200 classes. | ||
|
||
## Robust Critical Fine-Tuning | ||
|
||
### Demo | ||
|
||
Here we present a example for RiFT ResNet18 on CIFAR10. | ||
|
||
Download the adversarially trained model weights [here](https://drive.google.com/drive/folders/1Uzqm1cOYFXLa97GZjjwfiVS2OcbpJK4o?usp=drive_link). | ||
|
||
``` | ||
python main.py --layer=layer2.1.conv2 --resume="./ResNet18_CIFAR10.pth" | ||
``` | ||
|
||
- layer: the desired layer name to fine-tune. | ||
|
||
Here, layer2.1.conv2 is a non-robust-critical module. | ||
|
||
The non-robust-critical module of each model on each dataset are summarized as follows: | ||
|
||
| | CIFAR10 | CIFAR100 | Tiny-ImageNet | | ||
| -------- | -------------------- | -------------------- | -------------------- | | ||
| ResNet18 | layer2.1.conv2 | layer2.1.conv2 | layer3.1.conv2 | | ||
| ResNet34 | layer2.3.conv2 | layer2.3.conv2 | layer3.5.conv2 | | ||
| WRN34-10 | block1.layer.3.conv2 | block1.layer.2.conv2 | block1.layer.2.conv2 | | ||
|
||
### Pipeline | ||
|
||
1. Characterize the MRC for each module | ||
`python main.py --cal_mrc --resume=/path/to/your/model` | ||
This will output the MRC for each module. | ||
2. Fine-tuning on non-robust-critical module | ||
Based on the MRC output, choose a module with lowest MRC value to fine-tune. | ||
We suggest to choose the **middle layers** according to our experience. | ||
Try different learning rate! Usually a small learning rate is preferred. | ||
`python main.py --layer=xxx --lr=yyy --resume=zzz` | ||
When fine-tuning finish, it will automatically interpolate between adversarially trained weights and fine-tuned weights. | ||
The robust accuracy, in-distribution test acc are evaluated during the interpolation procedure. | ||
3. Test OOD performance. Pick he best interpolation factor (the one with max IID generalization increase while not drop robustness so much.) | ||
`python eval_ood.py --resume=xxx` | ||
|
||
## Results | ||
|
||
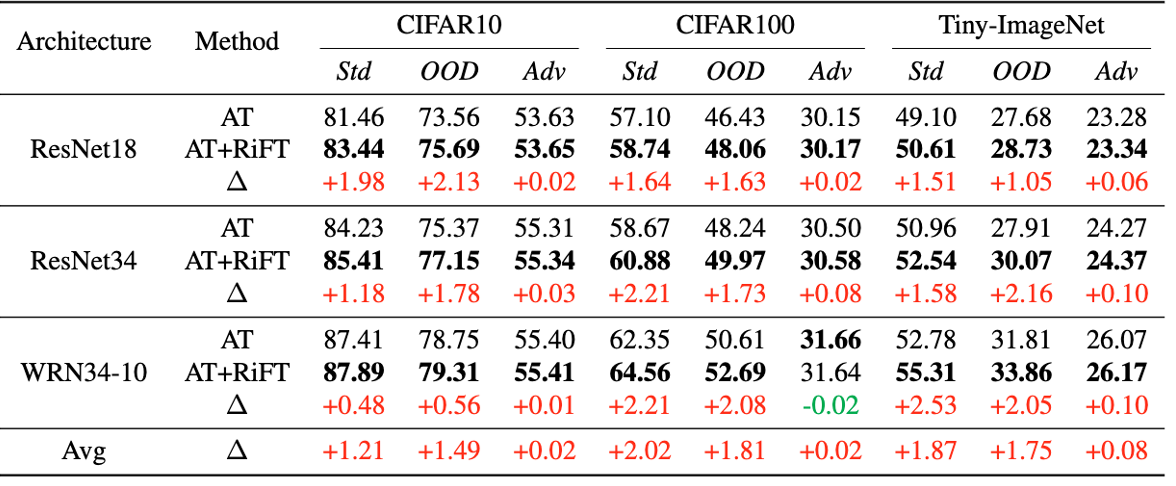 | ||
|
||
|
||
|
||
|
||
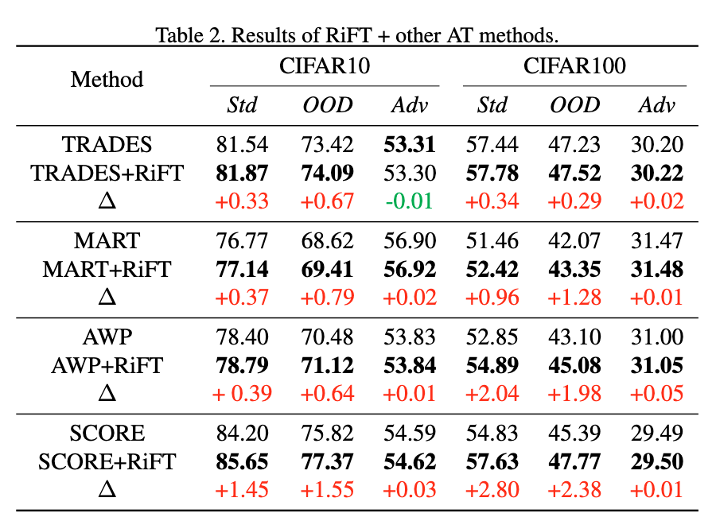 | ||
|
||
|
||
|
||
## References & Opensources | ||
|
||
- Classification models [code](https://github.com/kuangliu/pytorch-cifar) | ||
- Adversarial training [code](https://github.com/P2333/Bag-of-Tricks-for-AT) | ||
|
||
## Contact | ||
|
||
- Kaijie Zhu: [email protected] | ||
- Jindong Wang: [email protected] | ||
|
||
## Citation | ||
|
||
``` | ||
@inproceedings{zhu2023improving, | ||
title={Improving Generalization of Adversarial Training via Robust Critical Fine-Tuning}, | ||
author={Zhu, Kaijie and Hu, Xixu and Wang, Jindong and Xie, Xing and Yang, Ge }, | ||
year={2023}, | ||
booktitle={International Conference on Computer Vision}, | ||
} | ||
``` | ||
|
Oops, something went wrong.