-
Notifications
You must be signed in to change notification settings - Fork 5
Commit
This commit does not belong to any branch on this repository, and may belong to a fork outside of the repository.
Merge pull request #57 from alan-turing-institute/dj-module
update dj course with first module content
- Loading branch information
Showing
13 changed files
with
312 additions
and
40 deletions.
There are no files selected for viewing
Loading
Sorry, something went wrong. Reload?
Sorry, we cannot display this file.
Sorry, this file is invalid so it cannot be displayed.
Loading
Sorry, something went wrong. Reload?
Sorry, we cannot display this file.
Sorry, this file is invalid so it cannot be displayed.
Loading
Sorry, something went wrong. Reload?
Sorry, we cannot display this file.
Sorry, this file is invalid so it cannot be displayed.
This file contains bidirectional Unicode text that may be interpreted or compiled differently than what appears below. To review, open the file in an editor that reveals hidden Unicode characters.
Learn more about bidirectional Unicode characters
Original file line number | Diff line number | Diff line change |
---|---|---|
@@ -0,0 +1,33 @@ | ||
# What is data justice? | ||
|
||
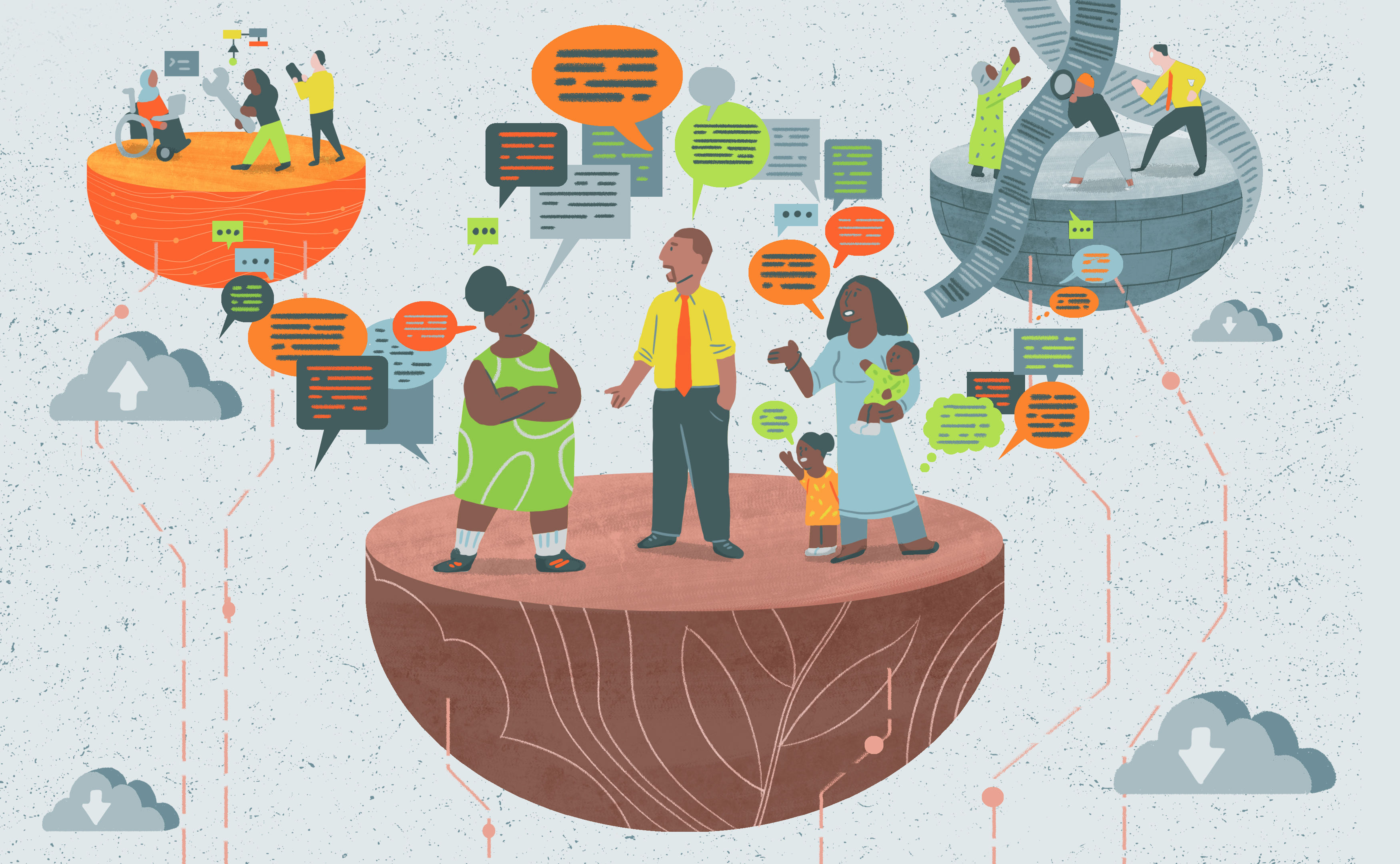 | ||
_‘Impacted Communities’ illustration by Johnny Lighthands, Creative Commons Attribution-ShareAlike 4.0 International_ | ||
|
||
Data-intensive technologies are increasingly deployed and used for diverse applications across domains, such as healthcare, policing, and education. | ||
Although such technological advances may offer various opportunities, there is a growing body of research and practice that highlights how the proliferation of data-intensive technologies exacerbate longstanding social inequities, or even contribute to the generation of new ones. | ||
|
||
As with any sociotechnical phenomenon, data-intensive technologies are neither neutral nor apolitical. | ||
They come into being through a mixture of human values, behaviours, and decisions of the creators of such technologies[^1]. | ||
Researchers have studied the role of social structures within and around data-intensive technologies across intersecting social dimensions, including class, race, and gender. | ||
They have shown that algorithms and systems of classification are necessarily shaped by historical patterns like socio-economic, racial, and gender disparities in technical professions, and other manifestations of discrimination in society, and that they could reinforce such patterns of inequality[^2]. | ||
|
||
!!! example "Illustrative example: Facial recognition technologies" | ||
|
||
The ways in which data is collected, processed, and used can have significant impacts on the outcome of the system whether it is assisting with the provision of social services or determining what videos you may want to watch based on your past viewing history. If data about certain groups are scarce, incomplete, or missing, this could have significant impacts on the overall output of a model. | ||
|
||
To illustrate the point, we can use the example of facial recognition technologies which are trained to recognise faces of individuals. In an example illustrated by Joy Buolamwini and Timnit Gebru[^3], a facial recognition classifier performed the worst on female faces with darker skin due to the underrepresentation of females with darker skin and individuals with darker skin in general in the datasets. | ||
|
||
Due to the lack of representation of women and darker-skinned women in the datasets, | ||
the classifier was more likely to fail to recognise their faces, leading to potential harms like wrongful arrests and negative stereotyping which could in turn reinforce historical patterns of discrimination towards these marginalised groups. In this instance, the dataset, often called the training set (a dataset used to train the model on past historical patterns) was unrepresentative and therefore led to harmful impacts. This illustrates how the ways in which this data is collected and the information it contains is critical and has real-world impacts on those for whom the outcome of the model is intended for. | ||
|
||
In response to such injustices reflected in data, there has been a growing movement of researchers, practitioners, and civil society groups seeking to address, challenge, and reimagine current practices of datafication. | ||
Data justice has emerged as a framework to characterise the multifaceted efforts to identify and enact ethical paths to social justice in an increasingly datafied world [^4]. | ||
|
||
For a quick recap of the emerging movement of data justice, take a look at the short infographic video below. | ||
|
||
<iframe width="560" height="315" src="https://www.youtube.com/embed/sgpnKbAZJvQ?si=RcRSARzUHf3JKxPa" title="YouTube video player" frameborder="0" allow="accelerometer; autoplay; clipboard-write; encrypted-media; gyroscope; picture-in-picture; web-share" referrerpolicy="strict-origin-when-cross-origin" allowfullscreen></iframe> | ||
|
||
[^1]: Winner, Langdon. 1980. ‘Do Artifacts Have Politics?’ Daedalus 109(1): 121–36. | ||
[^2]: Eubanks, V. (2018). Automating inequality: How high-tech tools profile, police, and punish the poor. St. Martin’s Press; Benjamin, R. (2019). Race after technology: Abolitionist tools for the new Jim code. Polity Books; D’Ignazio, C., & Klein, L. F. (2020). Data feminism. MIT Press. | ||
[^3]: Buolamwini, J., & Gebru, T. (2018, January). Gender shades: Intersectional accuracy disparities in commercial gender classification. In Conference on fairness, accountability and transparency (pp. 77-91). PMLR. https://proceedings.mlr.press/v81/buolamwini18a.html | ||
[^4]: Taylor, L. (2017). What is data justice? The case for connecting digital rights and freedoms globally. Big Data & Society, July-December, 1-14. https://doi.org/10.1177/2053951717736335 |
This file contains bidirectional Unicode text that may be interpreted or compiled differently than what appears below. To review, open the file in an editor that reveals hidden Unicode characters.
Learn more about bidirectional Unicode characters
Original file line number | Diff line number | Diff line change |
---|---|---|
@@ -0,0 +1,38 @@ | ||
# A brief history of data justice literature | ||
|
||
Before the emergence of a dedicated body of literature on the concept of data justice, responses to the increasing datafication of society tended to focus primarily on issues of data protection, privacy, and security [^1]. | ||
|
||
The first wave of data justice scholarship—emerging in the pathbreaking work undertaken by the [Data Justice Lab](https://datajusticelab.org/) at Cardiff University and the [Global Data Justice](https://globaldatajustice.org/) project at the Tilburg Institute for Law, Technology, and Society—sought to move beyond this limited view by situating the ethical challenges posed by datafication in the wider context of social justice concerns. | ||
This initial data justice research sought to be more responsive to the real-world conditions of power asymmetries, discrimination, and exploitation that have come to define the “data-society nexus” [^2]. | ||
|
||
!!! info "Data-society nexus" | ||
|
||
In “Exploring data justice: Conceptions, applications, and directions”, Dencik, Hintz, Redden, and Treré describe: | ||
|
||
“This shifts the focus of the data-society nexus away from simple binaries that frame the debate in terms of trade-offs or ‘good’ vs. ‘bad’ data in which data is an abstract technical artefact. Instead, data is seen as something that is situated and necessarily understood in relation to other social practices” (2019, p. 873). | ||
|
||
This first wave of data justice approached critical ethical questions primarily through a focus on surveillance, information capitalism, and the political economy of data. | ||
This focus on the political and economic forces surrounding datafication[^3], however, was less concerned with the underlying sources of data injustice linked with deeper socially, culturally, and historically entrenched structures of domination. | ||
Further to this, it has been noted that much of the academic discourse around data-intensive technologies has been dominated by global north perspectives, interests, and values[^4]. | ||
Approaches to data justice have yet a long way to go in incorporating and engaging with global majority visions of ethical and just ways of working, being, and interconnecting with people and the planet that are rooted in non-Western belief systems. | ||
|
||
The agenda of data justice aspires to encompass a sufficiently broad reach that recognises the plurality of ways of being and the living contexts of all individuals and communities impacted by datafication and digital infrastructures globally. | ||
For this reason, the inclusion of non-Western knowledges, world views and values that might shape possible data governance futures is a crucial precondition of advancing data justice research and practice. | ||
|
||
!!! example "" | ||
|
||
_From Shmyla Khan, Digital Rights Foundation_ | ||
|
||
Migrants and refugees are inherently vulnerable and precarious bodies, often occupying a liminal space within the imagination of body politic as well as the state. | ||
|
||
Speaking from the experience of Pakistan, surveillance, datafication, and exclusion of these bodies has been central to the nation-building process. Dealing with several waves of migrants, first after partition from British India and then the influx of migrant populations from newly independent Bangladesh provide good insight into the post-colonial national-building process. In the first wave it was integral to the nation that Muslims coming from across the newly-imposed Indian border be absorbed within the country, Pakistan Citizenship Act,1952 provides an expansive definition of who can claim to be a citizen. However, we see state practice change with the influx of migrants and displaced persons after the 1971 war, as Bihari migrants flowed in from Bangladesh. Many of these migrants still lack official citizenship and documentation despite having a strong claim of citizenship. Many of them are concentrated in informal settlements, with their families denied national identification to this day in 2022. They repeatedly face issues with registration into the National Database and Registration Authority (NADRA), unable to become data subjects in the eyes of the state. | ||
|
||
The third wave of migration in the country has been refugees from across the border with Afghanistan in wake of the Soviet invasion in the 1980s and has continued with the rule of the Taliban and US invasion. These refugees have been systemically denied citizenship, even when next generations have laid claim to legal birth right citizenship. However, the state has sought to look at these bodies from the prism of national security and surveillance--biometric Proof of Registration (PoR) cards are issued to refugees by NADRA. Despite being datafied, these bodies are still looked upon with suspicion—there are regular purging drives by NADRA to cancel registration of registration of documentation for refugees or anyone suspected of being Afghan. These bodies are coded as security risks, their informal settlements often razed to the ground on flimsy suspicions of crime -- always existing in that liminal space despite registration and datafication. | ||
|
||
[^1]: Leslie, D., Katell, M., Aitken, M., Singh, J., Briggs, M., Powell, R., Rincon, C., Chengeta, T., Birhane, A., Perini, A., Jayadeva, S., & Mazumder, A., (2022). Advancing data justice research and practice: An integrated literature review. http://dx.doi.org/10.2139/ssrn.4073376 | ||
|
||
[^2]: Dencik, L., Hintz, A., Redden, J., & Treré, E. (2019). Exploring data justice: Conceptions, applications, and directions. Information, Communication & Society, 22(7), 873-881. https://doi.org/10.1080/1369118X.2019.1606268 | ||
|
||
[^3]: Dencik, L., Hintz, A., & Cable, J. (2016). Towards data justice? The ambiguity of anti-surveillance resistance in political activism. Big Data & Society, 3(2), https://doi.org/10.1177/2053951716679678 | ||
|
||
[^4]: Aggarwal, N. (2020). Introduction to the special issue on intercultural digital ethics. Philosophy & Technology, 33(4), 547-550. https://doi.org/10.1007/s13347-020-00428-1; Mhlambi, S. (2020). From rationality to relationality: Ubuntu as an ethical and human rights framework for artificial intelligence governance. Carr Center Discussion Paper Series, 2020(009). https://carrcenter.hks.harvard.edu/files/cchr/files/ccdp_2020-009_sabelo_b.pdf; Birhane, A. (2021). Algorithmic injustice: a relational ethics approach. Patterns, 2(2), 100205. https://doi.org/10.1016/j.patter.2021.100205 |
Oops, something went wrong.